AI and Sustainability - Session I
- 12.10.23
- 10:00 - 12:00
- Chair : Grit Behrens and Kostas Karatzas
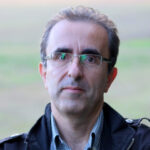
Reviewing explainable Artificial Intelligence towards better air quality modelling
- Kostas Karatzas
- Environmental Informatics Research Group, School of Mechanical Engineering, Aristotle University of Thessaloniki
- Hilpoltstein
- 10:00
The increasing complexity of machine learning models used in environmental studies necessitates robust tools for transparency and interpretability. This paper systematically explores the transformative potential of Explainable Artificial Intelligence (XAI) techniques within the field of air quality research. A range of XAI methodologies, including Permutation Feature Importance (PFI), Partial Dependence Plot (PDP), SHapley Additive exPlanations (SHAP), and Local Interpretable Model-Agnostic Explanations (LIME), have been effectively investigated to achieve robust, comprehensible outcomes in modeling air pollutant concentrations worldwide. The integration of advanced feature engineering, visual analytics, and methodologies like DeepLIFT and Layer-Wise Relevance Propagation further enhance the interpretability and reliability of deep learning models. Despite these advancements, a significant proportion of air quality research still overlooks the implementation of XAI techniques, resulting in biases and redundancies within datasets. This review highlights the pivotal role of XAI techniques in facing these challenges, thus promoting precision, transparency, and trust in complex models. Furthermore, it underscores the necessity for a continued commitment to the integration and development of XAI techniques, pushing the boundaries of our understanding and usability of Artificial Intelligence in environmental science. The comprehensive insights offered by XAI can significantly aid in decision-making processes and lead to transformative strides within the fields of Internet of Things and air quality research.
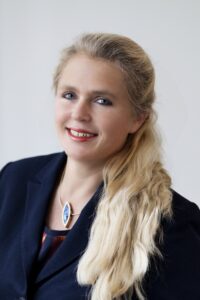
Commonalities and differences in ML-piplines for Air Quality Systems
- Grit Behrens
- Hochschule Bielefeld
- Hilpoltstein
- 10:30
This paper compares three ML-pipelines in Air Quality (AQ) Systems, namely a fog layer management model for IoT-systems, a low-cost AQ sensor system with sensor calibration and data fusion competences and a ML-method research based on low-cost OpenSensorMap. The three ML-pipelines are described, commonalities and differences worked out and the advantages of every technique are led over in an effort of a combined ML-pipeline which could be realised in a scientific cooperation of the three groups.
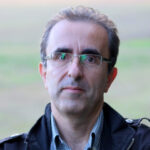
Optimal stacking identification for the machine learning assisted improvement of air quality dispersion modeling in operation
- Kostas Karatzas
- Environmental Informatics Research Group, School of Mechanical Engineering, Aristotle University of Thessaloniki
- Hilpoltstein
- 11:00
Air quality modeling plays a crucial role in understanding and predicting the dispersion of pollutants in the atmosphere, aiding in the development of effective strategies for mitigating the adverse impacts of air pollution. Traditional air quality modeling commonly relies on deterministic models that simulate pollutant transport, and dispersion based on physical and chemical principles leading to analytical numerical simulations towards the identification of pollutant concentrations in ambient air. However, these models often face challenges in accurately capturing the complex and dynamic nature of pollutant behavior due to uncertainties in emission inventories, meteorological conditions, and local-scale variations in terrain and land use. ENFUSER is a local scale air quality model that operates in the greater Helsinki area in Finland that successfully addresses most of the mentioned challenges. In previous research [2] we formalized a machine learning-based methodology to assist the operational ENFUSER dispersion model in estimating the coarse particle concentrations. Here, we continue this line of research and evaluate the genetic algorithm hybrid stacking with a novel validation procedure coined spatiotemporal cross validation. The development of the validation procedure was deemed necessary to simulate closely the operational requirements of ENFUSER. Furthermore, we introduce a fitness function based on robust statistics (median and standard deviation) that forces the predictions to follow the distribution of the reference stations. Results obtained using the greater Helsinki area (including Vantaa and Espoo) as a testbed suggest that the combination of ENFUSER with the proposed framework can provide estimations with higher confidence and improves the correlation from 0.61 to 0.71, the coefficient of determination from 0.34 to 0.50 and reduces the RMSE by 2.2 μg/m3.
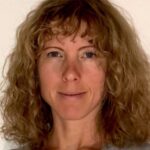
Concepts for Open Access Interdisciplinary Remote Sensing with ESA Sentinel-1 SAR Data
- Jennifer McClelland
- JKI-FLF
- Hilpoltstein
- 11:30
Earth observation with advanced, large-scale technologies as satellite piloted Synthetic Aperture Radar (SAR) appear essential to monitor agricultural ecosystems in the near future. Radar backscatter, for example, allows insights into crop conditions, soil properties, and direct mapping of vegetation growth. Precise SAR pre-processing is a substantial prerequisite to perform machine learning on SAR data, e.g., for early prediction of optimal sowing, harvesting, and fertilization time points. This is essential not only for successful, resource-efficient, and environmentally friendly farming but also for a wide range of other fields concerning environmental observations. Open access technologies offer the best solutions for collaborative efforts, thus minimizing financial and legal constraints in comparison to technologies residing in the commercial sector. Here, we combine expertise from the area of computer science, data science, software engineering, agriculture, and geo-information systems to build on state-of-the-art, open-source (OS) tools and technologies in Germany. Our goal is to provide an easy-to-employ Sentinel-1 SAR pre-processing tool as well as a Germany-wide, open access, pre-processed, analysis-ready database of Sentinel-1 SAR data. With the employment of modern software developing methods, including the Model View Controller (MVC) architecture and a procedural and object-oriented design, these solutions can be extended, adapted, and tested. This solution is available and accessible.