Keynote
- 11.10.23
- 11:15
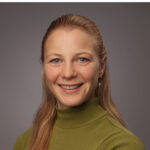
- Dr. Magdalena Mittermeier
- Department of Geographie, Ludwig-Maximilians-Universität München
- Sylvenstein
- Chair : Dieter Kranzlmüller
The available amount of data in climate sciences has been rapidly increasing in recent years, especially through a growing number of complex climate model simulations on a steadily finer spatial resolution. In particular, the generation of large ensembles of multiple climate model simulations contribute to Big Data in climate sciences through their high-complexity, high-velocity, and high-volume. Large ensembles are a valuable tool when analyzing climate impacts and the influence of natural climate variability on extreme events. This allows to distinguish between the effects of natural climate variability and anthropogenic climate change. The Big Data scale of large climate model ensembles calls for adequate methods for data and information processing. Deep Learning can be a helpful tool to analyze Big Data in climate sciences.
In this talk, the ClimEx (Climate Change and Extremes) Project is presented, which looks at the differentiation of natural climate variability and climate change impacts on hydro-meteorological extreme events on a regional scale. Europe was hit by several hydro-meteorological extreme events in recent years such as heavy precipitation and floods or on the other hand droughts. Summer floods in the northern alpine foreland are often triggered by a rarely occurring low-pressure system called Vb-cyclone (category 5, type B). Summer droughts, on the other hand, are often related to anticyclonic, sometimes long-lasting high-pressure conditions over Europe. Here, deep neural networks help to efficiently detect the weather patterns that trigger extreme events in atmospheric air pressure fields of large climate model ensembles. Once detected, their natural fluctuations and climate change impacts on their occurrence can be studied. Using these examples of the interaction of extreme weather conditions and hydrological extremes in the scope of the ClimEx-project, the benefits of using deep neural networks to address the Big Data scale of large climate model ensembles is illustrated.