Keynote
- 11.10.23
- 13:00
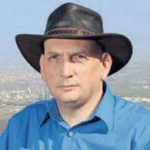
- Barak Fishbain
- Technion - Israel Institute of Technology Haifa
- Sylvenstein
- Chair : Volker Wohlgemuth
Mobile monitoring provides robust measurements of air pollution. However, resources constraints often limit the number of measurements, and thus these cannot be obtained in all locations of interest. To this end, surrogate measurement methodologies, such as videos and images were suggested. Previous studies of air pollution and images have used static images (e.g., satellite images or Google Street View images). The current study aimed at developing deep learning methodologies for inferring on-road pollutant concentrations from videos acquired by dashboard-cameras. Fifty hours of on-road measurement of four pollutants (black carbon, particle number concentration, PM2.5 mass concentration, carbon dioxide) in Bengaluru, India, were analyzed. The analysis of each 5-second video frame involved identifying objects and determining motion (by segmentation and optical flow). Based on these visual cues, a regression convolutional neural network (CNN) was used to deduce pollution concentrations. The findings showed that the CNN approach outperformed several other machine learning (ML) techniques and more conventional analyses (e.g., linear regression). The CO2 prediction model achieved a normalized root-mean-square error of 10% to 13.7% for the different train-validation division methods. The results here thus contribute to the literature by using video and the relative motion of on-screen objects rather than static images and by implementing a rapid-analysis approach enabling analysis of the video in real-time. These methods can be applied to other mobile-monitoring campaigns since the only additional equipment they require is an inexpensive dashboard camera.
Shot Bio: Barak Fishbain is an associate Professor at the Environmental, Water and Agricultural Engineering Division, Faculty of Civil & Environmental Engineering in the Technion-Israel Institute of Technology Haifa, Israel. Prior to his arrival to the Technion, Professor Fishbain served as an associate director at the Integrated Media Systems Center (IMSC), Viterbi school of engineering, University of Southern California (USC) and did his post-doctoral studies at the department of Industrial Engineering and Operations Research (IEOR) in University of California at Berkeley. Professor Fishbain’s research focuses on Enviromatics, devising AI and machine learning methods and mathematical models for better understanding built and natural complex environments. The goal is to harness new machine learning and mathematical models with engineering principles, computing, and networked sensing data analytics for enhancing the efficiency, resiliency, and sustainability of infrastructure and natural systems. This includes topics related to hydro-informatics, atmospheric-informatics, traffic data, structural health, smart infrastructure systems and connected transportation.